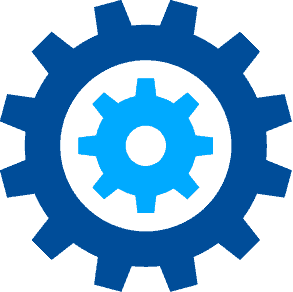
Written by Gimmal Product Marketing
In our current rapidly evolving technological landscape, enterprises are collecting, analyzing, and leveraging unprecedented amounts of data. This massive influx of data, coupled with the growing capabilities of Artificial Intelligence (AI), has made governance a critical aspect of modern organizations. However, the concepts of “AI Governance” and “Data Governance,” while interconnected, are often conflated or misunderstood. In this blog post, we will clarify the difference between these two governance frameworks, explore their synergies, and highlight how Gimmal can help organizations become AI-ready in their data governance journey.
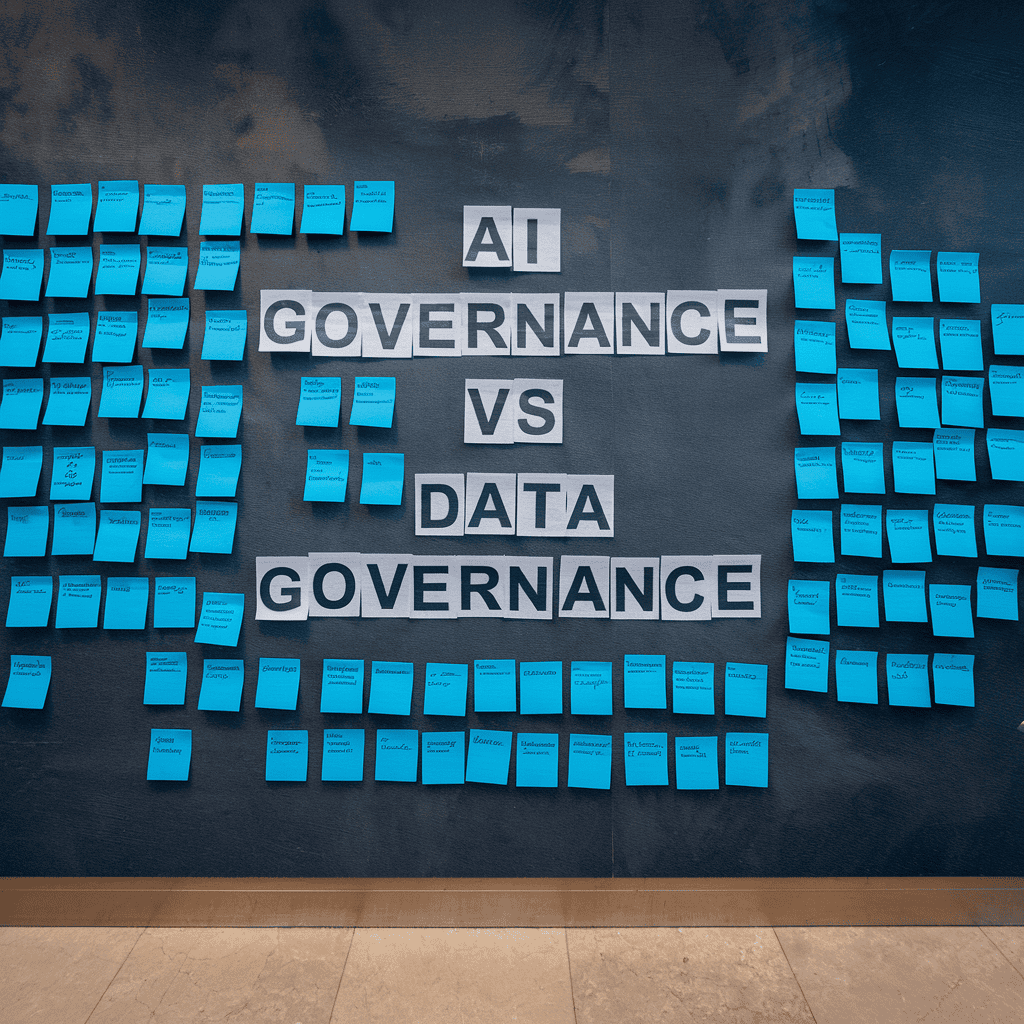
YouTube Video: What is AI governance?
What is AI Governance?
AI Governance refers to the framework of policies, processes, and practices that guide the development, deployment, and oversight of AI systems. It aims to ensure that AI-driven products and services are ethically designed, transparent, and aligned with organizational values, as well as societal norms and laws. Given the complexity and potential impact of AI systems, AI governance is crucial for mitigating risks such as bias, data misuse, intellectual property infringement, and more.
Key Components of AI Governance
1. Ethical and Responsible AI
Embedding fairness, transparency, and accountability into AI models and ensuring that automated decisions align with ethical guidelines.
2. Regulatory Compliance
Adhering to emerging AI regulations, standards, and guidelines (e.g., EU AI Act), along with industry-specific compliance requirements.
3. Risk Management
Identifying and mitigating risks associated with AI applications, including reputational risks, model performance issues, and potential biases.
4. Explainability and Auditability
Designing AI systems so their decision-making processes can be monitored, audited, and explained to stakeholders—especially crucial in regulated industries.
What is Data Governance?
Data Governance encompasses the processes, policies, and standards that guide how data is collected, stored, protected, and utilized within an organization. These policies ensure data accuracy, consistency, integrity, availability, and security. Effective data governance helps organizations manage data as a valuable asset—just like financial or human resources assets—and ensures that data is treated responsibly.
Key Components of Data Governance
1. Data Quality and Accuracy
Ensuring that data remains consistent, accurate, and fit for its intended purpose.
2. Data Lifecycle Management
Establishing policies and procedures to manage data throughout its lifecycle—from creation to archival or deletion.
3. Data Security and Privacy
Defining and enforcing protocols that protect data from unauthorized access while maintaining compliance with privacy regulations such as GDPR, CCPA, and HIPAA.
4. Data Stewardship
Assigning key roles and responsibilities for ensuring data quality, integrity, and security. Data stewards act as custodians to enforce governance policies.
How AI Governance and Data Governance Work Together
While data governance and AI governance address different aspects of an organization’s digital environment, they are highly interconnected. Effective AI governance is virtually impossible without robust data governance because AI systems rely on high-quality, well-governed data to produce reliable and ethical outcomes.
Data Quality Fuels Accurate AI
AI models depend on having large volumes of high-quality data. If data is poorly governed—riddled with errors, missing fields, or lacking context—AI outputs become unreliable and potentially harmful.
Privacy and Compliance
Both AI governance and data governance involve protecting users’ privacy and ensuring compliance with regulations. Robust data governance policies help ensure that data is used responsibly in AI processes.
Ethics and Bias Detection
AI governance frameworks look to detect and mitigate biases. Data governance ensures consistent standards for data collection and labeling, which can help diminish biases introduced by poor data quality or unrepresentative data sets.
Lifecycle Oversight
Both frameworks require organizations to maintain oversight throughout the data and AI lifecycles. From how data is collected and stored to how AI models are trained, deployed, and monitored, a cohesive approach is critical.
Achieving AI Readiness with Gimmal
Organizations looking to harness AI effectively must first ensure robust data governance practices. But where do you begin, and how can technology solutions like Gimmal help you get there?
Gimmal specializes in end-to end information lifecycle management, records management, and information governance. By offering a scalable platform to manage data end-to-end, Gimmal sets up organizations for success on their AI journeys. Here’s how:
1. Streamlined Content and Records Management
Gimmal’s unified approach to managing documents, records, and other information ensures all data is consistently categorized, stored, and secured. This foundation is critical for AI readiness, as AI systems rely on accurate label structures and metadata.
2. Compliance and Policy Enforcement
With powerful policy enforcement capabilities, Gimmal helps organizations comply with regulations while alleviating data sprawl. For AI use cases, this means easy traceability and auditability of data—key factors in ethical AI governance.
3. Enhanced Data Discoverability
Gimmal solutions provide search and classification tools that help you quickly find the data you need, which is invaluable for training AI models or discovering hidden insights.
4. Lifecycle Management and Disposition
AI governance depends on timely data updates and consistent oversight. Gimmal’s lifecycle management tools apply retention and disposal schedules in accordance with policies, ensuring that your AI training data is always current and compliant.
5. Seamless Integration
Gimmal integrates with various systems and repositories, allowing organizations to maintain a single source of truth. This consolidated view of data is immensely helpful for AI applications that require cross-departmental data.
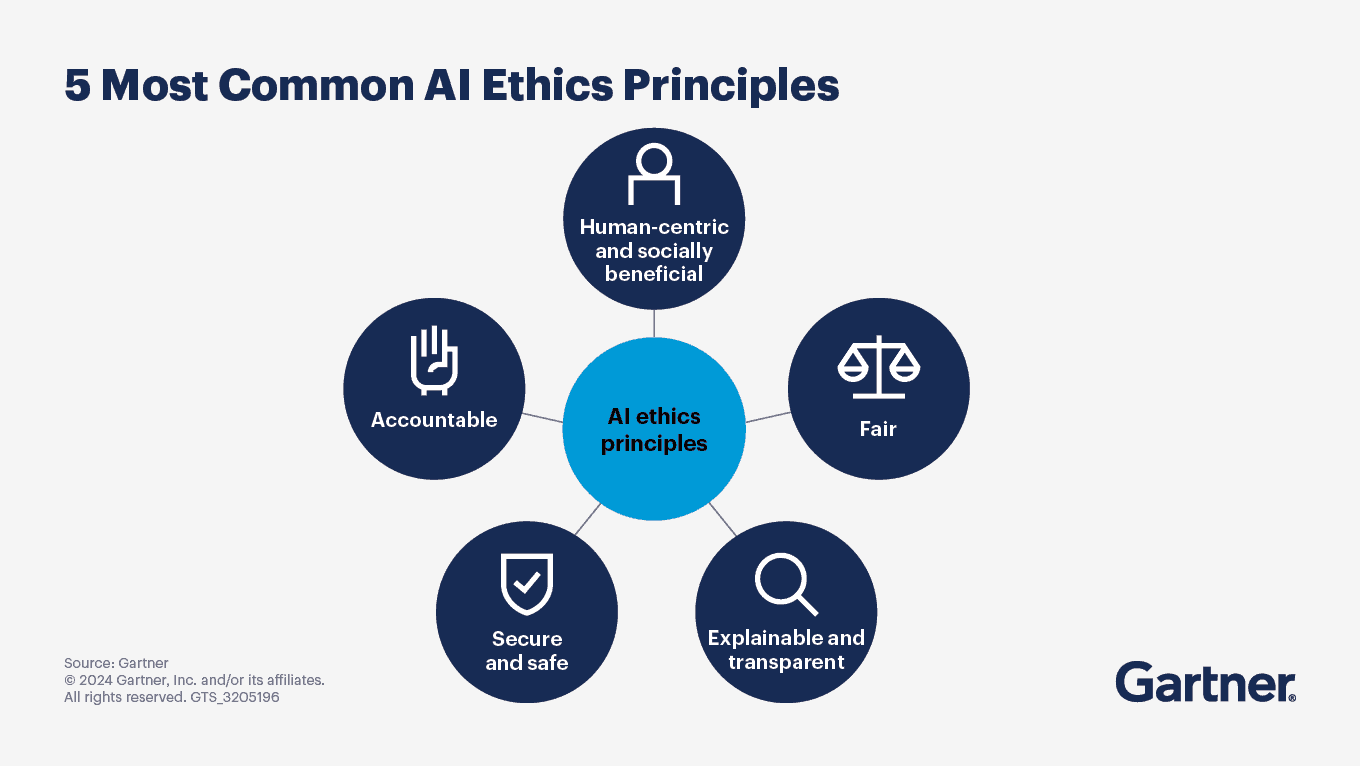
Conclusion
AI Governance and Data Governance are two sides of the same coin—both aim to ensure responsible use of data and technology in a world where information is abundant and the stakes are high. With robust data governance as a foundation, AI governance becomes more practical, ethical, and beneficial. Whether you’re looking to avoid biased AI models, safeguard consumer privacy, or comply with ever-changing regulations, aligning AI governance with data governance is essential.
By leveraging Gimmal’s information governance solutions, organizations gain a solid foundation upon which to build AI capabilities. Gimmal ensures data is trustworthy, accessible, and ethically managed, setting the stage for successful, forward-thinking AI implementations. When data governance is done right, AI governance becomes a natural extension—propelling your organization to effectively harness the transformative power of AI.
Ready to Learn More?
Get started by filling out the form below, and let us help you leverage your existing infrastructure with minimal disruption. Whether you’re looking for ease of use, a single platform solution, or guidance on information governance, we’re here to assist.
Related Content
Gimmal Records and RIMtech’s Catalyst: A Joint Records Management Solution
Accelerate your Records Mangement Implementation and Achieve Compliance Streamline your journey to an efficient Electronic Document/Records Management System (EDRMS) with a SharePoint-only solution featuring rapid deployment, pre-built information architecture, and a...
Data Retention Policies in the AI Era: What’s Changing?
Data Retention Policies in the AI Era: What’s Changing? Jan 16, 2025 The landscape of data management is undergoing a seismic shift as artificial intelligence becomes increasingly central to business operations. Organizations are now grappling with unprecedented...
Ready or Not: Building & Deploying AI-Ready Data
Ready or Not: Building & Deploying AI-Ready Data Dec 17, 2024 Artificial Intelligence (AI) is transforming industries at an unprecedented pace, offering organizations the potential to unlock new levels of efficiency and innovation. However, amid the excitement, a...
How to Avoid Writing RFPs and Simplify the Procurement Process
How to Avoid Writing RFPs and Simplify the Procurement Process Dec 10, 2024 Navigating the complexities of procurement can be overwhelming. If you’ve ever been part of the purchasing process, you’re likely familiar with the labyrinth of regulations,...
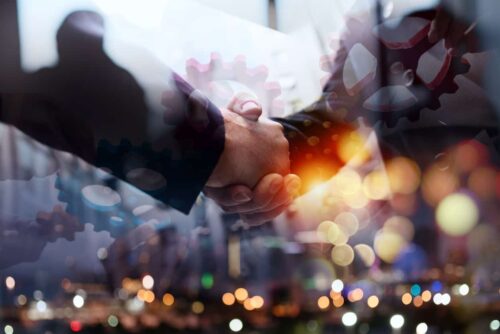
GRC Market Leader Gimmal Launches Comprehensive Legal Hold Solution with Microsoft 365 Integration for Content Preservation
HOUSTON, TX — February, 29, 2024 — Gimmal, the market’s only end-to-end information governance platform, announced the launch of its Legal Hold solution, a comprehensive and centralized Software-as-a-Service (SaaS) platform for streamlining complex legal...