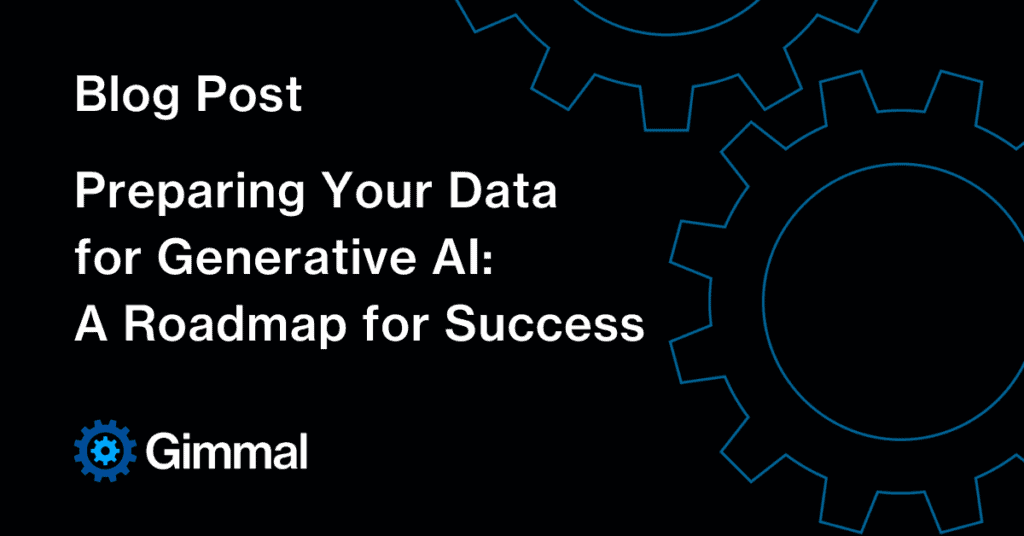
Generative AI (genAI) is poised to revolutionize how organizations operate, but the foundation of any successful AI initiative lies in the quality and accessibility of the data that fuels it. To unlock the full potential of genAI, businesses must ensure their data is not only comprehensive and relevant but also well-organized and secure.
However, the path to preparing data for AI is often fraught with challenges. Organizations frequently struggle with issues such as data quality, integration, and governance, which can hinder the effectiveness of AI initiatives. Gartner reported that by 2027, 80% of data and analytics (D&A) governance initiatives will fail due to a lack of a real or manufactured crisis.
So, how can organizations overcome these hurdles and get their data AI-ready?
The Importance of Data Readiness for AI
For generative AI to deliver meaningful insights and value, it requires access to a wide range of well-prepared data. Yet, most organizations are still grappling with fragmented data environments. A typical enterprise has data scattered across multiple repositories—both physical and digital—and in a mix of structured and unstructured formats. Without a clear strategy for organizing and preparing this data, businesses risk feeding their AI systems with incomplete or inaccurate information, as well as sensitive data, leading to suboptimal outcomes.
As Harvard Business Review highlighted in their 2024 report, “91% of survey respondents agree that an organization must have a reliable data foundation to successfully adopt artificial intelligence (AI)”.
The bottom line: Good data is the cornerstone of successful AI projects.
Steps to Prepare Your Data for AI
1. Conduct a Data Audit
The first step in preparing data for AI is to understand what data you have and where it resides. This requires a comprehensive audit of both physical and digital records. By creating a detailed inventory of your data assets, you can identify gaps, redundancies, and RIOT (Redundant, Inaccessible, Obsolete, and Trivial) data, as well as classify information according to its relevance to your AI use cases.
At Gimmal, we recommend leveraging automated data classification tools, such as our Sensitive Data Assessment (SDA) service, which scans and categorizes your data based on pre-defined criteria. The SDA tool also identifies sensitive data and RIOT data that may pose security risks or hinder AI performance. By identifying unnecessary or outdated data, the SDA service optimizes data quality and mitigates potential compliance issues. This not only speeds up the auditing process but also ensures that no critical information is overlooked, while streamlining the data environment for AI readiness.
2. Ensure Data Quality
Once you have a clear understanding of your data landscape, the next step is to improve its quality. Poor data quality—whether due to missing, outdated, or inaccurate information—can severely hinder the effectiveness of generative AI models. Every year, poor data quality costs organizations an average of $12.9 million, highlighting the significant financial impact of unclean data.
According to a 2022 report by Harvard Business Review, 47% of newly created data records have at least one critical error. These errors can propagate through AI systems, leading to flawed insights and decisions. Improving data quality through automated tools for data cleansing and validation can help ensure that your data is accurate, complete, and AI-ready. This process is not a one-time effort but an ongoing practice that businesses must integrate into their data management strategies.
At Gimmal, we offer solutions that help organizations maintain high data quality through automation and real-time validation, ensuring that your AI initiatives are built on a foundation of clean, reliable data.
3. Implement Comprehensive Data Governance
Data governance is crucial in ensuring that the information used in AI projects is accurate, secure, and compliant with relevant regulations such as GDPR, CCPA, and HIPAA. As AI becomes an increasingly integral part of business operations and data driven decision making becomes more prevalent, organizations need to establish robust governance frameworks to manage data quality, security, and compliance effectively.
One key aspect of data governance is aligning AI initiatives with existing privacy and compliance efforts. According to research from the International Association of Privacy Professionals (IAPP), 73% of organizations are leveraging their existing privacy expertise to manage AI governance. This approach ensures that AI models are built on properly governed data, which reduces the risks of non-compliance and enhances the effectiveness of AI-driven decision-making.
At Gimmal, we offer solutions that help organizations integrate strong data governance practices, ensuring that data is compliant, secure, and ready for AI applications. By applying consistent governance policies, businesses can confidently move forward in their AI journeys while mitigating risks.
4. Break Down Data Silos
Data silos are a major obstacle to AI-driven innovation. When data is locked away in isolated systems, it becomes difficult to access and analyze. To fully leverage genAI, businesses need to break down these silos and enable seamless data sharing across departments and systems.
By using platforms like Gimmal’s, organizations can create a unified repository for both structured and unstructured data. This not only makes it easier to feed comprehensive datasets into AI models but also facilitates collaboration and decision-making across teams.
5. Secure Your Data
As organizations move toward AI-driven initiatives, ensuring the security of their data becomes even more critical. The use of AI amplifies the potential risks associated with data breaches and misuse. Therefore, it’s essential to implement strong encryption, access control, and monitoring mechanisms to safeguard sensitive data.
According to IBM’s 2024 Cost of a Data Breach Report, the average cost of a data breach in the U.S. is now over $4.88 million per incident. Gimmal’s solutions are designed to protect your data throughout its lifecycle—from capture to destruction—using industry-leading security protocols.
Avoid Common Pitfalls
While the potential benefits of genAI are vast, there are several common mistakes that organizations make when preparing their data for AI-driven projects:
- Overloading the System: Attempting to feed too much data into an AI system at once can lead to inefficiencies. Start small by focusing on specific use cases with manageable data sets, then scale up.
- Ignoring Unstructured Data: A significant portion of enterprise data, such as emails, audio files, and PDFs, is unstructured. Ignoring this data can lead to incomplete AI insights. Ensure that your data preparation strategy includes methods for processing unstructured information.
- Assuming One-and-Done: Data preparation is not a one-time task. As new data is generated, it must be continuously evaluated, cleaned, and governed to maintain AI effectiveness.
Gimmal’s Approach to AI-Ready Data
At Gimmal, we understand the complexities involved in preparing data for AI. That’s why our solutions are designed to help organizations consolidate, govern, and secure their data in a way that maximizes its value for AI applications.
Our Gimmal Records platform, for example, allows organizations to automate records classification and retention, ensuring that only high-quality, relevant data is used in AI models. Additionally, Gimmal provides a unified platform for applying consistent governance policies across all data sources, reducing the risk of non-compliance and security breaches.
Reach out to us today if you’d like to learn more or schedule a demo.